Iza Discussion Paper Sarnikar Sorensen and Oaxaca Re Do You Receive a Lighter Prison Sentence Because You Are a Wi-oman 2007
Download original document:
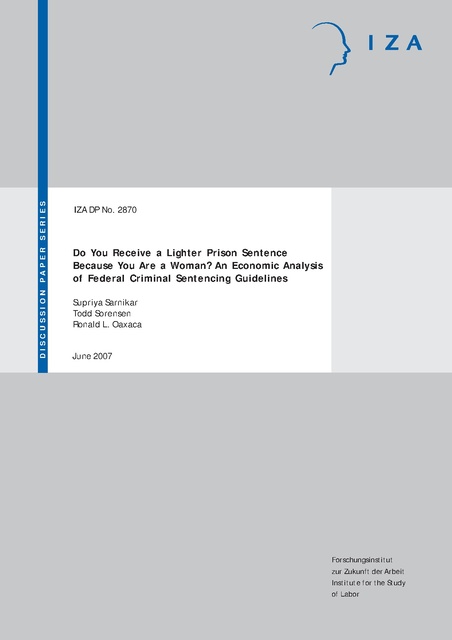
Document text
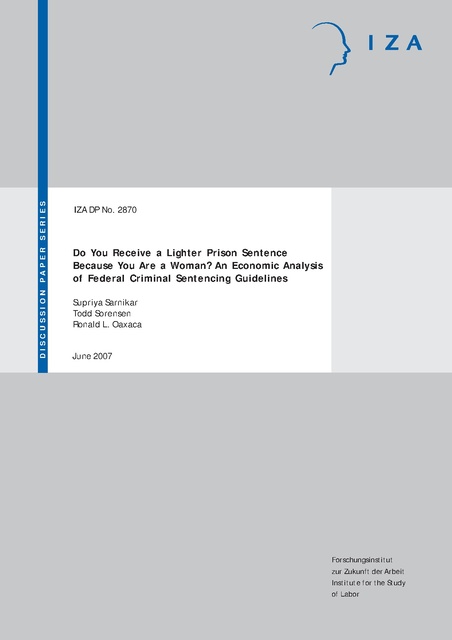
Document text
This text is machine-read, and may contain errors. Check the original document to verify accuracy.
DISCUSSION PAPER SERIES IZA DP No. 2870 Do You Receive a Lighter Prison Sentence Because You Are a Woman? An Economic Analysis of Federal Criminal Sentencing Guidelines Supriya Sarnikar Todd Sorensen Ronald L. Oaxaca June 2007 Forschungsinstitut zur Zukunft der Arbeit Institute for the Study of Labor Do You Receive a Lighter Prison Sentence Because You Are a Woman? An Economic Analysis of Federal Criminal Sentencing Guidelines Supriya Sarnikar Westfield State College Todd Sorensen University of Arizona and IZA Ronald L. Oaxaca University of Arizona and IZA Discussion Paper No. 2870 June 2007 IZA P.O. Box 7240 53072 Bonn Germany Phone: +49-228-3894-0 Fax: +49-228-3894-180 E-mail: iza@iza.org This paper can be downloaded without charge at: http://ssrn.com/abstract=999358 An index to IZA Discussion Papers is located at: http://www.iza.org/publications/dps/ Any opinions expressed here are those of the author(s) and not those of the institute. Research disseminated by IZA may include views on policy, but the institute itself takes no institutional policy positions. The Institute for the Study of Labor (IZA) in Bonn is a local and virtual international research center and a place of communication between science, politics and business. IZA is an independent nonprofit company supported by Deutsche Post World Net. The center is associated with the University of Bonn and offers a stimulating research environment through its research networks, research support, and visitors and doctoral programs. IZA engages in (i) original and internationally competitive research in all fields of labor economics, (ii) development of policy concepts, and (iii) dissemination of research results and concepts to the interested public. IZA Discussion Papers often represent preliminary work and are circulated to encourage discussion. Citation of such a paper should account for its provisional character. A revised version may be available directly from the author. IZA Discussion Paper No. 2870 June 2007 ABSTRACT Do You Receive a Lighter Prison Sentence Because You Are a Woman? An Economic Analysis of Federal Criminal Sentencing Guidelines* The Federal criminal sentencing guidelines struck down by the U.S. Supreme Court in 2005 required that males and females who commit the same crime and have the same prior criminal record be sentenced equally. Using data obtained from the United States Sentencing Commission’s records, we examine whether there exists any gender-based bias in criminal sentencing decisions. We treat months in prison as a censored variable in order to account for the frequent outcome of no prison time. Additionally, we control for the self-selection of the defendant into guilty pleas through use of an endogenous switching regression model. A new decomposition methodology is employed. Our results indicate that women receive more lenient sentences even after controlling for circumstances such as the severity of the offense and past criminal history. JEL Classification: Keywords: J78, K14, K42 discrimination, criminal justice, decomposition analysis, limited dependent variable analysis Corresponding author: Ronald L. Oaxaca Department of Economics University of Arizona McClelland Hall, Room 401QQ Tucson, AZ 85721-0108 USA E-Mail: rlo@email.arizona.edu * The authors gratefully acknowledge the helpful comments of participants at the 2006 Midwestern Economics Association meetings, the 2006 Western Economics Association meetings, and the 2006 WPEG conference at the University of Kent and of seminar participants at IZA. Gender equity has been one of the major global social issues to emerge out of the 20th century. A major focus of economists in this regard is on disparate labor market outcomes for men and women. Emphasis is placed on human capital explanations for gender wage gaps though there is some scope for other explanations, such as Becker taste-driven discrimination, statistical discrimination, and market power. Labor market outcomes potentially a¤ect future criminal activities, and criminal activities can a¤ect labor market outcomes. This paper examines the gender equity issue in the criminal justice arena and notes that labor market outcomes and criminal justice outcomes can be jointly determined. A popular perception in the criminal justice system is that female criminal behavior is a less serious problem than male criminal behavior. Detailed statistics compiled by the Bureau of Justice Statistics show that women commit fewer o¤enses than men and substantially di¤erent types of o¤enses than men. However, the statistics also reveal a rising trend in o¤enses committed by females and an increase in the incarceration of females in recent years. Beyond the labor market implications of gender equity in the criminal justice system there is also a concern for allocative e¢ ciency regarding resources devoted to deterrence and incarceration. The Federal Sentencing Guidelines that arose out of the Sentencing Reform Act of 1984 and that were subsequently struck down by the U.S. Supreme Court in 2005 (consolidated cases of United States v. Booker, No. 04-104, and United States v. Fanfan, No. 04-105) required that males and females who commit the same crime and have the same prior criminal record receive equal sentences. Critics of the sentencing guidelines argue that women should be accorded separate treatment because females who are caught in the criminal justice system “enter it due to circumstances that are distinctly di¤erent from those of men”1 . 1 Others argue that gender is not a factor “Research on Women and Girls in the Justice System.” National Institute of Justice Report (September 2000) at page iii. Available at http://www.ncjrs.gov/pd¢ les1/nij/180973.pdf. 2 that should enter into the sentencing decision. The Supreme Court in its split 5 to 4 decision argued that the mandatory guidelines violated the rights of criminal defendants to have a jury rather than a judge decide if defendants had committed all elements of a given crime. Consequently, the guidelines are only advisory to judges who may increase the length of sentences if they determine that the circumstances based on jury determination or admission of the defendant merit a longer prison sentence (Chicago Daily Law Bulletin 27 December 2005). The 2005 decision created some ambiguity in how far judges could stray from the now "advisory" sentencing guidelines. Currently, the Supreme Court is considering a case in which a sentencing judge departed from the guidelines, only to have an appellate court overrule the judge’s sentencing decision. The decision the Supreme Court hands down in this case may have as large an impact on the way sentences are given as the 2005 case2 (New York Times Feb 20, 2007) Whether the circumstances in which a crime is committed should be a consideration in criminal justice is not a question that we propose to answer here. Rather, we address the question of whether or not women do indeed receive more lenient sentences despite the sentencing guidelines. The answer to this question is important to both sides of the debate. Those in the justice system who favor equal treatment but believe that women are let o¤ too lightly may be especially harsh when judging a female accused of crime, while those who favor separate treatment of women but believe that they are treated equally may be less stringent. Thus, perceptions of unequal treatment, when they are not based on systematic study and sound facts, may lead to actual inequality in the justice system. A systematic study of whether bias actually exists is therefore not only necessary but timely given the rising trend in o¤enses committed by women and the increase in female incarceration rates as 2 The pending cases are Clairborne v. United States, No. 06-5618 and Rita v. United States, No. 06-5754. 3 evidenced by the data compiled by the Bureau of Justice Statistics. Further work can begin to better tie the relationship between gender equity in the criminal justice system with gender equity in the labor market. An unpublished paper by Oaxaca and Sarnikar (2005) [henceforth OS] uses a rich data set on sentencing outcomes from the United State Sentencing Commission to estimate separate logistic regressions for men and women, where the dependent variable is a binary variable measuring whether or not convicted individuals received federal prison time. While summary statistics from their data set show that females are less likely to receive prison time than males, more sophisticated analysis can take account of covariates that can explain some or all of the gender sentencing di¤erential. In this paper, we consider outcomes from the sentencing process more broadly for a sample of whites who were convicted while the mandatory sentencing guidelines were still in e¤ect. Speci…cally, we look beyond the binary Prison/No-Prison outcome to a continuous measure of prison sentence. Ideally, one would want to take into account the fact that defendants must choose whether or not to plea bargain or to take their chances in a trial. Given that we work with a sample of convicted individuals (we do not have data on acquittals), we model the probability of whether the conviction was the result of a trial versus a plea bargain. We treat sentences handed down due to guilty pleas as outcomes from one regime, while sentences given to defendants convicted in a trial are treated as outcomes from a separate regime. This approach allows characteristics to be weighted di¤erently depending on the path to conviction. One would expect that the average sentences of identical defendants facing identical charges should be lower in the plea regime.3 In our data set, around 25% of all criminal sentences involve no prison time. Because of this considerable mass point at 3 If the sentences were lighter in the trial regime it would be di¢ cult to believe that defendants would ever do anything but plead not guilty, as this would generate a positive probability of facing no sentence at all. 4 zero, it may be inappropriate to consider the distribution of sentencing outcomes to be continuous. Also, the plea vs. trial regime is a choice variable for the defendant so that we must account for self selection in our model. Accordingly, we treat the outcome variable as a mixed discrete continuous variable. Therefore, our econometric model is a censoring (Tobit) switching regression with endogenous switching, which we estimate by full information maximum likelihood (FIML). To measure how much of the male/female sentencing di¤erential can be attributed to di¤erences in the characteristics of men and women, compared to how much of the di¤erential can be explained by di¤erences in the weights applied to these characteristics by judges, we develop a new decomposition. This decomposition builds upon Neuman and Oaxaca (2004), which addresses the issue of selectivity in the context of a Heckman sample selection model. We expand this analysis to decompose di¤erentials in the switching regression model with censoring. Our approach takes account of the fact that predicted outcome means will not generally match sample outcome means because of the highly non-linear nature of the model. Within our data set, the scarcity of observations on females and the preponderance of observations in the plea regime conspire to leave us with an insu¢ cient sample size to properly apply FIML to estimate the sentencing determination model separately for females. In the decomposition developed here, we exploit an insight from Oaxaca and Ransom (1994) that allows us to decompose the male-female regime and sentencing di¤erentials without actually estimating the model for females. Rather than comparing weights from a male only and female only model, we instead are able to compare the estimated parameters from the model for males with parameters estimated in a pooled model for males and females. 5 LITERATURE Since the seminal work of Becker (1968), there has been a signi…cant amount of research aimed at understanding the economics of crime. In the basic economic model of crime, a rational individual decides whether or not to allocate his/her time to criminal activity by comparing the expected net return from criminal activity to the expected returns from legitimate activity. The expected net return to criminal activity consists of the potential …nancial and psychic bene…ts (B) of committing the crime minus the cost (C) of committing the crime. The cost to the individual of committing the crime is determined as the product of the probability (p) of being caught by law enforcement and the severity of the punishment (S). If the returns to legitimate labor market activity is the wage (W), then a rational, risk-neutral individual will engage in criminal activity only if B-pS > W. This static model therefore predicts that criminal activity can be deterred by either increasing the probability of detection(p), the severity of punishment(S) and/or the wage rate (W) in the labor market. Economists have since subjected these theoretical predictions to empirical testing using econometric models of varying degrees of sophistication. Ehrlich (1973) and Levitt (1997) estimate the impact of increased law enforcement presence on crime and …nd that increasing law enforcement e¤orts have the desired e¤ect of lowering the incidence of crime. Ehrlich (1977) estimated the deterrence e¤ect of capital punishment on crime. Witte (1980) …nds that the deterrence e¤ect of higher legal wages was small compared to the deterrence e¤ects of the severity and certainty of state imposed penalties. Johnson, Kantor and Fishback (2007) study the e¤ect of social insurance on crime rates. Block and Gerety (1995) reports on laboratory experiments that examine di¤erences between the criminal population and the general population in the relative responsiveness to the deterrence e¤ects of severity of punishment versus the deterrence e¤ects of the certainty of punishment. The results showed that convicts 6 were more deterred by increases in the certainty of punishment whereas the student subjects were more deterred by increases in the severity of punishment. Kuziemko (2006) uses New York State’s reinstatement of the death penalty to identify the effect of capital punishment on plea bargaining outcomes. Freeman (1999), Grogger (1998), and Gould, Weinberg and Mustard (2002) …nd that falling real wages were a signi…cant determinant of increasing crime rates during the decades of the 1970s and 1980s. The link between deterrence e¤orts and crime rates is an endogenous one. Decisions to increase law enforcement e¤orts are often made in response to increasing crime rates. Similarly, di¢ culty in …nding legitimate labor market employment might push some individuals into criminal activity but the fact that an individual has engaged in criminal activity also would lower that individual’s probability of …nding legitimate employment. Myers (1983) investigates whether poor labor market prospects postrelease a¤ect the re-integration of ex-convicts into the mainstream. Using di¤erent data sets, Myers …nds that better wages post-release signi…cantly reduced recidivism. Witte and Reid (1980) also …nd that receiving a high wage on the …rst job after being released from prison decreases recidivism and that the wage rate received by a prison ‘releasee’ depends mostly on the demand side characteristics such as the industry and occupation rather than on the accumulated human capital of the ‘releasee’. Imai and Krishna (2004) estimate a dynamic model of criminal behavior and show that expected future adverse consequences in the labor market prove to be an e¤ective deterrent to crime. Waldfogel (1994) estimated the e¤ects of conviction and imprisonment on post-conviction income and employment probabilities and found that the state-imposed sanctions were much smaller in comparison to the “market sanction”, which he estimated as the income lost due to conviction and imprisonment. Also, the “market sanction” was signi…cant only for those o¤enders who worked at jobs that required much trust. Grogger (1995) used longitudinal data and concluded that the 7 strong negative correlation between arrests and subsequent labor market sanctions that was found in earlier cross-sectional studies was largely due to unobserved characteristics that in‡uence both criminal and labor market behavior. Grogger (1995) however does …nd that there are signi…cant negative consequences of arrests in the labor market but that they are short-lived. Consistent with the predictions of the economic model of crime, the Sentencing Reform Act of 1984 (SRA 1984) increased the length of punishment for almost all crimes, eliminated probation and reduced the possibility of parole for good behavior. Kling (2006) estimates the e¤ect of this increased severity of punishment on labor market prospects of criminals post-release. Kling …nds that there is no signi…cant adverse e¤ect on employment or earnings of criminals due to longer incarceration lengths and concludes that this may be because prison rehabilitation programs may be o¤setting the loss of potential work experience and human capital depreciation while in prison. The sentencing guidelines formulated pursuant to the SRA 1984 aimed to provide uniform sanctions for the same crime by eliminating gender, age, or racial disparities in sentencing. While economists have studied the deterrence e¤ect of severity of punishment quite extensively, relatively little literature exists on the optimality and desirability of uniform sentencing. Lott (1992) argues against uniform sentencing based on the …nding that market sanctions in the form of lost incomes, opportunity costs of imprisonment and the adverse impact of incarceration on labor market prospects are disproportionately higher for individuals with higher incomes. Since the expected total monetary penalty includes the reduction in legitimate earnings capability post release, Lott argues that the state-imposed punishments should be proportionately adjusted. Moreover, since mere conviction can restrict the post-conviction opportunities for higher income individuals more severely than for lower skilled people, Lott argues that in order to equalize the severity of punishment, higher income individuals 8 would have to be convicted much less frequently than low-income criminals. One could argue that such equalization could be addressed by di¤erential sentence length. However, the sentencing guidelines explicitly prohibited sentencing judges from considering factors such as the defendant’s socioeconomic status, race, sex, age, and religion. The punishment was to be proportional to the severity of the crime and the defendant’s criminal history alone. Judicial discretion to change the sentence based on characteristics of the defendant was thus severely restricted under the guidelines. Several studies in the criminology literature have examined gender and racial disparities in sentencing prior to the formulation of sentencing guidelines. See Tonry (1996) for a survey of these studies. Whether the guidelines have been successful in reducing the disparity has also been studied extensively both in the criminology and the law and economics literature. Anderson, Kling and Stith (1999), Kempf-Leonard and Sample (2001) study sentencing disparities before and after the federal sentencing guidelines. Mustard (2001) looks at racial and gender disparities in sentencing under the federal guidelines and …nds that observed disparities in sentencing are mainly due to the special circumstances when judges are allowed to depart from the guidelines and not due to discriminatory tastes of judges. Schanzenbach (2005) estimates the e¤ect of judicial demographics on sentencing outcomes and …nds that increasing the proportion of female judges increases the gender disparity in sentencing and interprets this as evidence that male judges are paternalistic and therefore lenient towards female o¤enders. Almost all of the studies mentioned infer gender-based discrimination in sentencing from the statistically signi…cant coe¢ cient on a dummy variable indicating the gender of the criminal o¤ender. Yet, sentencing discrepancies may be observed merely because a judge takes into account extralegal circumstances of the defendant. If the circumstances of male and female criminal defendants are substantially di¤erent, as claimed by several authors, then the consideration of circumstances by judges may 9 appear as gender-based bias even when the judge exhibits no such discriminatory tastes. Verdier and Zenou (2004) show that when there is statistical discrimination in the labor market and everyone believes that blacks, for example, are more likely to engage in criminal activity, then such beliefs lead to lower wages for blacks. When the opportunity cost of a crime is thus lowered, such beliefs become self-ful…lling and lead to higher crime rates among blacks. It is therefore important to thoroughly investigate whether any bias actually exists in the criminal justice system since perceived bias may itself lead to actual bias. Given the adverse labor market consequences of incarceration, unequal treatment of men and women in the criminal justice system may lead to unequal prospects for men and women in the labor market as well. Our research design separates the e¤ect of di¤erences in circumstances from the e¤ect of di¤erences in weights attached to circumstances by judges. If a judge attaches di¤erent weights to the same circumstances of a male and a female o¤ender, then we may attribute that to a gender-based bias. But if a judge attaches the same weights to circumstances but on average awards di¤erent sentences to male and female o¤enders then that di¤erence in sentencing might be due to di¤erences in circumstances of the two defendants. Oaxaca and Sarnikar (2005) use decomposition analysis to investigate whether there exists any leniency towards women in the binary decision of whether or not to imprison a convicted person. The results of this decomposition show that the di¤erences in characteristics explain more than 100% of the gender sentencing gap. If, when determining whether or not to sentence a woman to prison, judges applied the same weights on characteristics as they use for men, women would actually be slightly less likely to face prison. DATA The data used in this study are obtained from the United States Sentencing Commission’s data collection e¤orts and pertain to cases that terminated in convictions 10 over the period 1996-2002. The data set is available from the Federal Justice Resource Statistics Center. In order to abstract from sentencing issues associated with race and ethnicity, we have con…ned our attention to convicted white males and white females. There were a total of 45,060 sentencing cases in our sample (37,104 cases for males and 7,956 cases for women). Table 1 presents a summary of the share of sentences involving no prison time. Overall, a higher percentage of females receive no prison time upon conviction. This is true for both the trial and guilty plea regimes. For both males and females, conviction by a guilty plea is associated with a larger percentage of sentences involving no prison time. The variables reported in Table 2 are the ones we have constructed for use in our sentence determination model. Both the measure of …nal o¤ense level and the criminal history variable are set according to a …xed formula. To calculate the o¤ense level, the case is assigned a base level for o¤ense and then adjusted for various aggravating circumstances such as the use of a …rearm in the crime or obstruction of justice, or for mitigating circumstances such as acceptance of responsibility. The criminal history measure is a function of both the length of prior imprisonments and how recently these sentences were given.4 . While men on average are awarded longer prison sentences (42 months) than women (17 months), the severity of their o¤enses as measured by the …nal o¤ense level scores are greater on average than those of women. Also, men on average have a higher past criminal history score than women. Convicted men are on average two years older than convicted women and are more likely to have private counsel. A higher percentage of men are college graduates (13% vs. 7%). 4 For details on their construction of these variables, please see the following documents on the USSC’s website: http://www.ussc.gov/training/sent_ex_rob.pdf http://www.ussc.gov/training/material.htm 11 In Table 3 we present summary statistics pertaining to the average length of sentences imposed on both men and women in each of our sample years. Note that in each year the average male sentence is more than twice that of the average female sentence. If one were to only consider these summary statistics and no covariates, it would appear that women receive considerably lighter sentences than do males, and that this di¤erence is considerably greater in the trial regime. Overall and in the trial regime, average male sentences generally declined over the sample period while average female sentences actually rose. Average sentences in the plea regime tended to rise for both males and females. ECONOMETRIC MODEL Below we describe the econometric methods used to estimate the necessary parameters to decompose the sentence di¤erentials. First, we describe the model we use to decompose the sentence di¤erence into an explained portion (di¤erences in characteristics) and an unexplained portion (di¤erences in weights). Sentencing In our data set, we observe the sentencing outcomes for defendants whose cases reach the sentencing phase. Recall that there are two ways in which a defendant’s case can reach the sentencing phase. While a signi…cant number of defendants faced sentencing after being convicted by a jury, the most frequent way a defendant reached the sentencing phase was by pleading guilty. Plea bargains reached with a prosecutor are often the reason for this guilty plea; these defendants are sentenced under what we call the plea regime. When a defendant pleads not guilty, but is convicted in a trial, they are sentenced under the trial regime. We de…ne y as the months in prison the defendant is sentenced to, X as the vector of the individual’s characteristics, and 12 as the vector of weights on the defendant’s characteristics in the respective regimes. Equation (1) represents sentencing outcomes when an individual pleads guilty or is convicted by trial: yi = XP i XT i P T + "Pi if defendant is in plea regime + "Ti if defendant is in trial regime. (1) Although the formal model permits di¤erences in the covariates appearing in each sentencing regime, the empirical speci…cation actually used in this paper restricts covariates to be identical in both sentencing regimes. The very nature of a plea bargain suggests that the process determining the sentence of the defendant will not be the same in the two regimes. We would then expect the sentences received by two otherwise identical defendants to depend upon the way in which they reached the sentencing phase. Put another way, the weights applied to an individual’s characteristics will be di¤erent depending on which sentencing regime the defendant is facing. Accordingly, it may be inappropriate to pool observations from individuals in these two regimes into a single sentencing equation. If individuals were exogenously selected into one of the two regimes, we could simply estimate the two models separately. In order to more formally take account of the regime outcome conditional upon conviction, let P represent the probability of a guilty plea, ability of going to trial and being convicted, and T &A T &C represent the prob- represent the probability of going to trial and being acquitted. Conditional upon prosecution, these probabilities sum to 1. Because we do not have observations on those who went to trial and were acquitted, we can only estimate the following conditional probabilities: P = and T &C ; which sum to 1 and where P C is the + T &C P + T &C probability that one’s conviction was from a guilty plea and T C is the probability PC TC = P that one’s conviction was by trial. Let the variable s represent the conditional latent variable corresponding to a defendant’s conviction by trial. The variable s takes on 13 a value of 1 if the defendant’s conviction is by trial, and a value of 0 if the defendant enters a guilty plea. The vector index variable Zi is a set of variables a¤ecting this probability. Accordingly, the binary regime determination model may be expressed as si = Zi + ui 1 if si > 0 si = 0 if si 0: (2) (3) Correlation between unobservables in the plea decision stage and unobservables in the sentencing stage will create non random selection that will prevent us from obtaining consistent estimates of the parameters if they are estimated by OLS or Tobit. To account for this self-selection, we model the sentence determination process using a switching regression model with endogenous switching. We assume that the error term from each regime’s sentence determination equation follows a bivariate normal distribution with the error term from the selection equation. The nature of this model requires that an explicit distributional assumption be made. The structure of the error terms is given in the following variance-covariance matrix, where T denotes the trial regime, P denotes the plea regime, and s denotes the binary selection equation (the variance of which is normalized to 1)5 : 0 1 1 Ps Ts B C B C 2 V = B Ps PT C P @ A Ts 5 PT (4) 2 T The errors in the two sentencing regimes could be correlated; however the model neither requires nor provides identi…cation of this parameter. 14 The likelihood function of the model is then: N Y 1 yi XT i T L = Pr(ui > T i=1 si Zi j"T i ) T yi 1 XP i P P 1 si Zi j"P i ) Pr(ui P (5) This expression is simpli…ed once we take account of the conditional distribution of u on " : L = N Y i=1 ( ( 1 P 1 yi XT i T Zi + T Ts T 1 T yi XP i (yi Zi P Ps P 1 P XT i T) Ts (yi XP i P) Ps !)si !)1 si (6) One additional econometric problem we face is the non-continuous distribution of the dependent variable. Because sentence length cannot be negative, and nearly 25% of our sample receives no prison time, it may be necessary to account for this mass point at 0 in order to obtain consistent estimates.6 In the context of our switching regression model, we treat the dependent variable as a mixed discrete continuous variable, with limit observations at 0. The sentence outcome is now represented as yP i = XP i P + "Pi if defendant is in plea regime yP i if yP i > 0 and si = 0 yP i = 0 if yP i 0 and si = 0 yT i = XT i T + "Ti if defendant is in trial regime yT i if yT i > 0 and si = 1 yT i = 0 if yT i 0 and si = 1 6 (7) (8) (9) (10) We also estimate the model without accounting for censoring; the log-likelihood obtained is signi…cantly lower than that obtained in the model where we account for the censoring. 15 The likelihood for the switching regression with endogenous switching and censoring allows four di¤erent types of entries to the likelihood function: limit and non-limit observations in both of the regimes. The likelihood function is L = N Y 2 XTi ( ; Zi ; T i=1 ( s i li T 1 yT i T 1 P XT i T 2 Ts XP i Zi + Ts T (yT i 1 Zi P 2 XT i ; Zi ; T) Ts Ps P 1 P where l = 1 for limit observations and (1 si )li P P T yP i XP i (yP i Ps XP i Ps !)si (1 P) !)(1 li ) si )(1 li ) (11) represents the cumulative bivariate normal distribution. DECOMPOSING SENTENCING DIFFERENTIALS To examine how much of the gender di¤erence in sentences is due to leniency toward one sex or the other, we apply empirical methods developed in the labor economics literature to estimate gender bias in criminal sentencing outcomes. These meth- ods have the advantage of decomposing gender di¤erences in sentencing outcomes into two di¤erent components –one due to di¤erences in observable circumstances of males and females convicted by the criminal justice system and another due to differences in unobserved circumstances or attitudes of judges towards the sexes. Such decomposition is achieved by a three-step analysis. The …rst step typically involves estimation of our empirical model for males and females where the dependent variable is the length of the prison sentence. Here, instead of estimating the empirical model separately for both males and females, we estimate the model for males only. This approach is consistent with viewing the unexplained gap as a residual. It is also necessary in our case, as the relatively small 16 number of female observations in the trial regime means that we are unable to identify a number of parameters in an estimation of the model for females only. This approach allows us to decompose the di¤erential without estimating the female weights, thus circumventing the problem. Our analysis departs from previous studies in the second step and adds greater insight into the decision-making process that might lead to gender-based di¤erences in criminal sentencing. In the second step, we predict the average sentence length for females if they faced the male weights. In the third and …nal step, we use results from the …rst two steps and decompose the di¤erences in length of sentences for males and females into two components: one attributable to male-female di¤erences in circumstances and a second attributable to unobserved di¤erences in attitudes of judges towards the sexes and unobserved di¤erences in circumstances. Decomposition methods such as the one described above were …rst developed in labor market studies of gender and racial wage di¤erences [(Oaxaca 1973)] but have not been used in studies of gender or racial bias in criminal sentencing decisions. Such a method of estimating bias is valuable since it not only estimates any gender-based di¤erences in sentencing outcomes but it also identi…es whether the observed bias is due to gender di¤erences in circumstances or due to gender-based di¤erences in weights attached to circumstances by judges. In addition to the problems with identifying the female weights, we face two additional challenges which force us to expand beyond the Oaxaca (1973) decomposition. The issue of selection bias in decompositions is addressed by Neuman and Oaxaca (2004) in the context of a Heckit model. We are able to build o¤ of this work in the decomposition we develop, as the Heckit is essentially a special case of an endogenous switching regression model. Finally, we must account for the existence of the limit observations in our data set. 17 Decomposing Sentencing Outcomes by Regime First, consider the sentence determination equation for the trial regime: yT i = XT i T + "T i if defendant is in the trial regime yT i if yT i > 0; si = 1 yT i = 0 if yT i 0; si = 1 (12) (13) The expected value of a sentence in the trial regime is derived in Appendix 1. De…ne the sample average sentence in the trial regime as yT m for males and yT f for females. The sample is composed of NT m men and NT f women. The average predicted value of sentences for males is de…ned as: ybT m NT m 1 X = ybT mi ; NT m i=1 (14) where ybT mi is the predicted sentence for the ith male in the trial regime. However, in a …nite sample the predicted mean and the sample mean terms will not necessarily be equal, i.e. ybT m NT m NT m 1 X 1 X = ybT mi 6= yT m = yT mi in general. NT m i=1 NT m i=1 Assuming that the underlying model can be consistently estimated, we would have plim(b yT m yT m) = 0 (15) plim(b yT f y T f ) = 0: (16) When the predicted mean outcome does not match the sample mean outcome, we have sample mean prediction error. The proportionate sample mean prediction errors for males and females can be expressed as 18 It follows from consistency that bT m = y T m ybT m bT f = y T f : ybT f y plim(b) = plim yb (17) (18) = 1: Appendix 2 contains a more detailed discussion of the use of sample mean error predictions in the nonlinear decompositions adopted in this paper. The average value of sentences for females in the trial regime using male weights is de…ned as: y^T0 f = Nf X y^T0 f i i=1 (19) NT f where y^T0 f i is a …tted value of the ith female sentence had they faced the male weights. We decompose the di¤erence in average sentences in the trial regime as follows: yT m y T f = bT m (b yT m ybT0 f ) + (bT m bT f )b yT0 f + bT f ybT0 f ybT f : (20) The …rst term in eq (20 ) measures the explained sentencing gap while the unexplained gap is the sum of the last two terms. Note that the second term measures the contribution of gender di¤erences in the sample mean prediction error while the last term measures the contribution of gender di¤erences in the estimated parameters of the model.7 It is therefore possible to separate out the e¤ect of gender di¤erences 7 Of course there are many instances in which there is no discrepancy between sample means and predicted sample means, e.g. the linear regression model with a constant term, the logit model with a constant term, and the second stage regression of a heckit sample selection model. 19 in ^T if the econometrician estimates both bT m and bT f . While we are able to decompose the di¤erence in outcomes into the portion caused by di¤erences in weights and di¤erences in characteristics, we will be unable to isolate the di¤erence caused by weights into a portion caused by di¤erent ^T terms. However, if it is the case that bT m bT m yb0 Tf bT f 0, the unexplained gap is totally captured by bT f ybT0 f ybT f ybT f . Under these circumstances one could identify the predicted mean i 1 h 0 0 b outcome for females as ybT f ybT f yT m yT f bT m ybT f : Tm y bT m The decomposition of sentences in the plea regime follows closely that of the trial regime. Now using male weights from the plea regime, the …tted value of the length of sentence in the regime becomes y^P , which di¤ers slightly in form from y^T .8 Decomposing Regime Choice Now consider a decomposition of regime choice. Consider the regime determination model given in (2) and (3) where a positive outcome indicates conviction by trial. The observed proportion of females and males going to trial are, respectively pT f = pT m = Nf X sf i i=1 (21) Nf N m X smi i=1 (22) Nm We de…ne the di¤erence in outcomes for males and females as the observed di¤erences in proportions of males and females in the trial regime, pT m pT f . Recall that we do not estimate the model separately for females. 8 However, we The …tted value is now for individuals who are "selected in" in the plea equation, rather than the "selected out" observations in the conviction by trial equation. The form of the selectivity term will di¤er slightly. See Appendix 1 for the expressions governing the calculations of the mean outcomes. 20 are still able to decompose the di¤erence in male and female outcomes into the portion caused by di¤erences in characteristics and the portion caused by di¤erences in weights. We go about these single model decompositions by decomposing di¤erentials using only the estimated weights for males. Here, we decompose the di¤erence in the propensity of males and females to be convicted by trial regime using only male weights. Consider the regime determination model estimated for males: smi = Zmi m + ui 1 if smi > 0 smi = 0 if smi 0 (23) (24) The estimated weights in this model allow us to obtain a predicted probability of conviction by trial for each individual in the sample: p^T mi = (Zmi ^ m ) (25) We compute the average predicted probability by averaging the individual predicted probabilities: p^T m Nm X (Zmi ^ m ) = Nm i=1 (26) Note that in the probit model, unlike the logit model, the average predicted probability of entering the trial regime will not necessarily equal the proportion of the sample who do in fact enter the regime (for further work on the decomposition of di¤erentials in the context of a probit model, see Fairly (2005) and Yun (1999)). In practice the di¤erence is typically negligible. However, the selection probability parameters in our model are obtained from FIML applied to the joint estimation of the selection probability and sentencing equations. Hence, there is a need to scale the mean predicted probabilities when conducting a decomposition of gender di¤erences 21 in the propensity to be convicted via the trial regime. As above for the sentencing outcomes, the sample mean (probability) prediction errors for males can be expressed as follows: bsm = pT m p^T m (27) The same consistency argument applies here as in the case of sentencing outcomes. We estimate the average predicted probability of females being in the trial regime had they faced the same weights as the males: p^0T f Nf Nf X X p^0T f i (Zf i ^ m ) = = Nf Nf i=1 i=1 (28) The di¤erence in the average probability of conviction via the trial regime can then be decomposed as follows: pT m pT f = (pT m bsm p^0 ) + (bsm p^0 Tf Tf pT f ) (29) where the …rst term on the right hand side represents the di¤erence in probabilities that can be attributed to di¤erences in characteristics, and the second term represents the part of the di¤erence that can be attributed to di¤erences in weights. Total Decomposition Consider an algebraic decomposition of sentencing di¤erences by regime. De…ne ym as the average sentence for males in our sample, and yf as the average sentence for females. Each gender’s average sentence will be a weighted average of the average sentence in the two regimes: ym = yT m pT m + yP m (1 yf = yT f pT f + yP f (1 22 pT m ) pT f ) (30) (31) The di¤erence in average sentences can then be expressed as ym yf = yT m pT m + yP m (1 pT m ) yT f pT f Adding and subtracting the terms yT f pT m and yP f (1 yP f (1 pT f ) pT m ), and collecting terms appropriately yields ym yf = (yT m +(yT f yT f ) pT m + (yP m yP f ) (pT m yP f ) (1 pT m ) pT f ): (32) The …rst two terms in (32) can be interpreted as a weighted average of the di¤erences in mean sentence outcomes for men and women (weighted by the probability of being in each of the two regimes). The …nal term can be interpreted as the di¤erence in mean sentence outcomes that can be attributed to gender di¤erences in the propensities of being in the trial regime (weighted by the di¤erences in mean outcomes among females in the two regimes). Recall how we decomposed each of the single decomposition terms. Denote the portion of the di¤erence attributed to di¤erences in characteristics (the explained portion) as E. The portion of the di¤erence attributed to gender di¤erences in the parameters (the unexplained portion) is denoted as U . Each portion also contains a subscript denoting the part of the estimation from which it originates: 23 yT m yP m yT f = yP f i h ybT0 f ) + (bT m h bT m (b yT m = ET + UT h = bP m (b yP m i h ybP0 f ) + (bP m = EP + UP pT m bsm p^0 ) + (bsm p^0 Tf Tf pT f = (pT m bT f )b yT0 f + bT f ybT0 f ybT f bP f )b yP0 f + bP f ybP0 f ybP f i i (33) (34) pT f ) (35) = Es + Us The decomposition of the overall gender sentencing gap can then be expressed as ym yf = [(ET + UT ) pT m + (EP + UP ) (1 +(yT f pT m )] (36) yP f ) (Es + Us ) = ET pT m + EP (1 | +UT pT m + UP (1 | pT m ) + Es (yT f {z E pT m ) + Us (yT f {z U yP f ) } yP f ) ; } where E is the total amount of the overall gender sentencing gap that is explained by di¤erences in characteristics, and U is the total unexplained gap associated with di¤erences in weights. We note that a more straight forward total decomposition of the mean sentencing di¤erences between men and women can be calculated as ym yf = ym where y^f0 = P i ^m y^0 + (^m y^0 f f p^0T f i y^T0 f i + 1 Nf (37) yf ) p^0T f i y^P0 f i and ^m = P i [^ pT mi y^T mi + (1 Nm 24 p^T mi ) y^P mi ] 1 ym : In this decomposition y^f0 is the mean …tted overall sentence for females using the male weights. Empirically, it turns out that both (36 ) and (37) yield virtually identical values of the total explained and unexplained portions of the overall gender sentencing gap. However, a shortcoming of the decomposition given by (37) is that it obscures the sources of the overall gender sentencing gap revealed by the more detailed decomposition given in (36). RESULTS Formal theory does not o¤er very much guidance on the actual speci…cation of the regime selection and sentencing equations. The sentencing guidelines largely con…ned federal court judges to considering only current o¤ense level and criminal history when passing sentence. Speci…cally, the guidelines exclude race, sex, national origin, creed, religion, and socioeconomic status. Furthermore, employment and family ties and responsibilities are also not to be considered in awarding criminal sentences. With only limited exception, age and education are not supposed to be relevant for sentencing decisions. Judges are permitted to award lighter prison sentences to elderly defendants. Since we have data on these various potential factors, we are able to empirically determine the extent to which they turn out to in‡uence sentences because of, or despite, the guidelines. The variables that appear jointly in the regime selection and sentencing equations are indicators for females (in the pooled sample), education, marital status, the circuit court district, and year while the continuous variables appearing jointly pertain to prior criminal history, number of dependents, and age. An indicator for U.S. citizenship appears in the regime selection equation but not in the sentencing equations. While judges should not take into account the nationality of a defendant when determining her sentence, citizenship should serve as a proxy for this defendant’s knowledge of and experience with the U.S. criminal justice system; we would expect risk averse individuals with less knowledge of how 25 this process works to be less likely to take their chances in a trial rather than striking a plea bargain deal. An indicator for a defendant’s …ne being waived appears in the sentencing equation but not in the regime selection equation. This variable serves as a crude proxy for income. Also, a cubic polynomial function of the severity of the …nal o¤ense level appears in the sentencing equations but are excluded from the regime selection equation. Both the …ne variable and the …nal criminal o¤ense level variables are not determined at the time that the individual makes the decision about going to trial. Given that defendants do not have perfect foresight, these variables should determine the …nal sentence given but not a¤ect the plea decision. Although our data span both cases and years, it is not treated as a panel. The data are available as separate cross-sections by case for each year. Each case corresponds to all prosecutions ending in convictions of an individual in the given year and the total prison time awarded. While it is theoretically possible for an individual to appear in more than one year’s cross-section, we suspect that this is not very common. Among males the average prison sentence is 3.5 years over a period of 7 years. This does not leave much time for multiple year convictions unless o¤enses are committed while the individual is in prison. In the case of females the average prison sentence is 1.4 years over the period of our study. This would allow for multiple year convictions except that the crime rate is still much lower for females. Female cases account for just under 18% of the total number of cases in our data set. To get a sense of whether or not there may be favoritism towards women, we …rst estimate our model on a pooled sample of males and females, including an indicator variable for whether the observation is that of a female o¤ender. In Table 4 we present parameter estimates from this pooled sample of males and females The estimated coe¢ cient on the female indicator variable is negative and signi…cant in the selection equation, indicating that women are less likely to obtain their convictions via the trial regime, where average sentences are higher. More educated and married individuals 26 are more likely to obtain their convictions through trial rather than through guilty pleas. Being a U.S. citizen is associated with a lower probability of obtaining one’s conviction via trial as opposed to a guilty plea. The chances that one would obtain their conviction via trial rather than via a guilty plea rise with age until around 73 years after which the trial regime probability declines. The circuit court district in which the conviction took place does a¤ect the probability of conviction via trial vs. guilty plea. The year indicators (where 2002 is the omitted reference group) suggest that the probability of obtaining conviction via trial relative to guilty plea steadily declined over time. A more extensive past criminal history was positively associated with conviction by trial vs. a guilty plea. Having a private defense counsel has a statistically signi…cant negative impact on the probability of conviction by trial. The estimated coe¢ cients on the female gender indicator are negative and statistically signi…cant in both sentencing regimes, but they are of a greater magnitude (in absolute value) in the trial regime. Even before we allow all weights to di¤er by gender, this indicates that women may receive lighter sentences than men. This would seemingly violate the sentencing guidelines. Contrary to the guidelines, marital status and number of dependents do a¤ect prison sentences, but only in the plea regime. Married defendants receive shorter sentences in the plea regime. Having more dependents leads to shorter sentences in the plea regime. Age and education exhibit some e¤ect on sentences though ordinarily these are not considered relevant by the guidelines. Sentence length rises with age and peaks at 69 years if one is convicted in the trial regime and peaks at 29 years in the plea regime. Although the guidelines permit lighter sentences for the elderly, a peak of 29 years in the plea regime and the strong signi…cance of the age terms in the trial regime would not seem to be entirely consistent with the guidelines. Education appears to lower sentences in the plea regime and raise them in the trial regime. Those who have been convicted and had …nes waived receive longer sentences in the plea regime. If this variable adequately proxies 27 incomes of the defendants, then it would seem that poorer defendants receive longer sentences in the plea regime. As expected the extent of a defendant’s criminal history and severity of current …nal criminal o¤ense contribute to longer prison sentences in both regimes. The signs and magnitudes of the linear, quadratic, and cubic terms jointly imply that, for all relevant values of the variable, as the severity of the crime for which one is convicted increases, sentence length increases at an increasing rate. Having a private defense counsel lowered prison sentences in both conviction regimes.9 Similar to the case with conviction regime selection, the circuit court district in which the conviction took place does a¤ect sentence lengths. The estimated coe¢ cients on the time indicator variables reveal that, ceteris paribus, sentence length had been declining over time in the trial regime while rising in the plea regime. Estimates of the correlations between the conviction regime error and the sentencing regime errors suggest that unobservables in the selection equation are negatively correlated with unobservables in the trial sentencing equation and positively correlated with unobservables in the plea regime. Roughly speaking, this means that those who are more likely to select into the conviction by trial regime can expect shorter sentences in the trial regime and longer sentences in the plea regime. While this is a sensible result, one potential problem is that the estimated correlation coe¢ cient between the regime selection equation error term and the plea regime sentencing error term is close to the boundary value of 1. It is probably the case that this extreme estimate of the correlation coe¢ cient is caused by the fact that ninety …ve percent of the sample 9 If the choice of defense counsel and the conviction regime are jointly determined, then the choice of defense counsel would be endogenous in the model. Accordingly, we estimate a model to determine if the decision to be represented by a private attorney is jointly determined with regime choice. By estimating the model with a bivariate probit, we can test for this possible correlation in the two error terms related to these decisions. Our estimation …nds the error term correlation coe¢ cient to be insigni…cant, suggesting that the coe¢ cient on the defense counsel variable in the main model is consistently estimated. 28 represent convictions via guilty pleas. In Table 5 we report the FIML estimates based on just the male sample. Since the results for males are qualitatively the same as those for the pooled sample, we do not separately discuss these estimates. The major purpose behind estimating the model separately for males is to provide us with the necessary parameter estimates to compute the decomposition of gender di¤erences in prison sentences. Decomposition results are reported in Tables 6 through 8. We begin with Table 6 which presents mean sentencing outcomes by regime and regime selection di¤erences as well as predicted outcomes using estimated male weights. On average men are awarded nearly 25 more months of prison than women. This varies by sentencing regime. For those convicted by trial, men received an average of 69 more months of prison than women. Among those who plead guilty, men received an average of almost 22 more months of prison time than women. A higher percentage of men than women received their convictions via trial vs. a guilty plea, 5.5% vs. 3.5%. From the …tted (predicted mean) sentences for males, we are able to calculate the proportionate mean sample prediction errors. The most accurate prediction corresponds to the plea regime which is the one into which the vast majority of the cases fall. The last column of Table 6 reports the predicted outcomes for females using the FIML estimated weights for men and are comparable to the calculated …tted values for men reported in the next to the last column in Table 6. For the actual decompositions, the proportionate mean prediction errors for men are applied to the predicted outcomes for women obtained using the estimated male weights. The …gures in Table 6 clearly imply that if females had faced the same sentence determination process as men, they would have experienced longer prison sentences in each regime, though still less than those of men, and would have had a higher propensity to have received their convictions from the trial regime as opposed to the plea regime. Our decompositions of gender sentencing di¤erences in each regime and gender 29 di¤erences in conviction regime probabilities are reported in Table 7. Di¤erences in the female mean characteristics explain 46% of the gender sentencing di¤erential in the trial regime and 66% of the sentencing di¤erential in the plea regime. We observe that of the 69 month sentencing gap that favors women in the trial regime, nearly 38 months of the gap cannot be accounted for by gender di¤erences in circumstances. Of the 22 month sentencing gap that favors women in the plea regime, 7 months of the gap cannot be accounted for by gender di¤erences in circumstances. Only about 21% of the 2.1 percentage point gender gap in the propensity to obtain conviction in the trial regime can be explained by gender di¤erences in characteristics. Females are also less likely to be sentenced in the trial regime, though their characteristics suggest they would actually be more likely to be sentenced in this regime if they were to face the male weights (though still less likely than males). In Table 8 we parse out the components that add to the overall gender sentencing di¤erence across both conviction regimes. These components weight the explained and unexplained portions of the sentencing gaps in each regime by the probabilities of being in each regime and gender di¤erences in these probabilities. Of the nearly 25 month overall gender sentencing gap favoring women, 3.8 months (15.4%) arises from gender sentencing di¤erences in the trial regime. Gender sentencing di¤erences in the plea regime account for a little over 20 months (81.6%) of the overall gap. The remainder of less than one month (3.0%) is accounted for by gender di¤erences in conviction regime probabilities. Overall, the explained portion of the gap accounts for about 15.4 months (62.7%) of the total gender sentencing di¤erence. This leaves about 9.5 months (38.3%) that cannot be explained by gender di¤erences in circumstances. Table 8 disaggregates the explained and unexplained portions of the overall sentencing gap by contributions from each sentencing regime and sentencing regime probabilities. The plea regime accounts for the largest contribution to the overall explained gap (13.5 months or 87.6%) and to the overall unexplained gap (6.8 months or 72.0%). In fact 30 the largest single component of the constituent parts of the overall gender sentencing gap is the 13.5 month explained gap from the plea regime which accounts for 54.0% of the overall advantage of women in awarded sentences. CONCLUSION Unlike any studies in the literature so far, our study separates observed gender differences in sentencing into two di¤erent components –one attributable to di¤erences in circumstances of male and female criminal defendants, and the second attributable to di¤erences in attitudes of sentencing judges towards male and female defendants and the di¤erences due to unobservable characteristics of the male and female defendants. Our model takes account of the joint determination of sentences by regime and conviction regime selection as well as censoring occasioned by sentences that do not involve prison time. We are able to determine the role of gender di¤erences in selection regime probabilities. Such decomposition provides a better insight into the decision-making process of sentencing judges. Knowing whether judges consider extralegal circumstances in their decision making is important, but knowing how they consider extralegal circumstances is useful to policy makers in deciding how to reform sentencing guidelines to ensure equal treatment. This study not only examines whether judges consider extralegal circumstances but if they do, it asks whether they attach the same weight to circumstances of males and females. Even in light of the Supreme Court’s decision in 2005 to strike down the Federal Sentencing Guidelines, our results may o¤er some guidance as to what to expect now that judges are less constrained in imposing sentences. We …nd that women receive prison sentences that average a little over 2 years less than those awarded to men. Even after controlling for circumstances such as the severity of the o¤ense and past criminal history, women receive more lenient sentences. Approximately 9.5 months of the female advantage cannot be explained 31 by gender di¤erences in individual circumstances. In other words if women faced the same sentencing structure as men, women would on average receive 15.4 months less prison time than men rather than 24.9 months less prison time. Most of the gender gap arises from convictions via guilty pleas, which account for the vast majority of the convictions observed in our data. Besides gender, we …nd evidence that judges took into account factors such as family circumstances which are expressly prohibited from consideration when awarding sentences. One should bear in mind that our data permit us to examine only the end stage of the criminal justice system. A more comprehensive treatment would take account of the fact that before arriving at the judge for sentencing, a defendant must also pass through a jury or possible plea bargain with a prosecutor. Before reaching this stage, other groups, such as the police and the prosecution, have the potential to create bias in the criminal justice system. Future work will focus on separating out di¤erential outcomes layer by layer, as well as making explicit the impact of gender bias in the criminal justice system on gender di¤erences in labor market outcomes. 32 Appendix 1: Expected Value of Dependent Variable with Censoring The expected value of a censored dependent variable is simply the product of the probability of observing a non-limit observation and the expected value of the dependent variable given that it is a non-limit observation, plus the probability of observing a limit observation times the expected value of the dependent variable given that it is a limit observation. Because the censoring point is at zero, the expected value of limit observations is 0, causing the second term to drop from the expression. We …rst consider the trial regime: E[yT i jsi = 1] = Pr(yT i > 0jsi = 1) E[yT i jyT i > 0 \ si = 1] + Pr(yT i 0jsi = 1) E[yT i jyT i 0 \ si = 1] = Pr(yT i > 0jsi = 1) E[yT i jyT i > 0 \ si = 1] + Pr(yT i 0jsi = 1) 0 = Pr(yT i > 0jsi = 1) E[yT i jyT i > 0 \ si = 1] Consider each of the two right hand size terms separately. (1) First, consider the probability of observing a non-limit observation, conditional upon selection. From our speci…cation of the data generating process for y and s, we can express this as the function of two random variables, " and u. Pr(yT i > 0jsi = 1) = Pr("T i < XT i T jui < Zi ) (2) By Bayes’rule we can express this as the joint probability that a non-limit observation is selected into the trial regime, divided by the probability of that observation being 33 in the trial regime. This term can then be expressed using values from the cumulative normal and cumulative bivariate normal distributions. Pr( "TTi < Pr(yT i > 0jsi = 1) = 2( = XT i T T XT i T T \ ui < Z i ) Pr(ui < Zi ) ; Zi ; sT ) (3) : (Zi ) Finally, we must consider the expected value of the dependent variable, given that it is a non-limit observation in the trial regime. Recall that non-limit observations take on the value E[yT i jyT i > 0 \ si = 1] = E[yT i jyT i > 0 \ si = 1] = E[yT i jyT i > 0 \ si > 0] XT i T "T i < \ ui < Z i ] : = E[yT i j T (4) T This expected value appears similar to the expected value of the dependent variable in the main equation of the Heckit model: it is truncated by the draw for the error term in the selection equation. It also appears similar to the expected value of the dependent variable in the Tobit model: it is truncated by the draw for the error term in the main equation. This incidence of "double truncation" however, is substantially more complex than the single truncation in either the Tobit or the Heckit. We derive it for our model based on page 72 of Johnson and Kotz (1972): XT i E[yT i jyT i > 0 \ si = 1] = ( + Tf sT ( 2( XT i T T XT i T T T ; Zi ; 1 ) (p 1 1 ( Zi ) ( p 1 34 sT ) 2 sT 2 sT [ Zi [ XT i XT i T T T ]) ) ( Zi )]) (5) The resulting expected value of the length of sentence in the trial regime is: E[yT i jsi = 1] = 2( XT i T T ; Zi ; (Zi ) sT ) E[yT i ] We can then de…ne the y^T (X; Z; ^m ) = E[yT i ] as given above. 35 (6) Appendix 2: A Note on Sample Mean Prediction Error in Decompositions In decomposition analysis, the standard term to decompose is the di¤erence between the sample mean of the dependent variable for two groups. De…ne the sample mean values for groups m and f as y m and y f , where each group has Nm and Nf members, respectively. After estimating an econometric equation for both of the groups, we can then calculate …tted values ybmi and ybf i for each individual in groups m and f , respectively. The average …tted value for members of these groups is: ybm ybf Nm 1 X = ybmi Nm i=1 (1) Nf 1 X ybf i = Nf i=1 (2) De…ne ybfo i as the …tted value of an observation in group f , had that individual faced the group m estimated parameters. The mean of this variable for group f is then: ybf0 Nf 1 X 0 = yb Nf i=1 f i (3) By adding and subtracting the ybf0 term, the decomposition is then expressed as: ym ybf0 ) + (b yf0 y f = (y m yf ) (4) where the …rst term expresses the di¤erence in the left hand side variable which can be attributed to di¤erences in the characteristics of the two groups, and the second term expresses the di¤erence caused by di¤erences in the parameters the two groups face. Assuming that the underlying model can be consistently estimated, we would have 36 plim(b ym ym) = 0 (5) plim(b yf yf ) = 0 (6) However, in a …nite sample, the yb and y terms will not necessarily be equal. We can express the sample mean prediction error in the model as follows: y m = bm ybm It follows from consistency that y f = bf ybf plim(b) = plim The decomposition can now be expressed as: ym (7) y f = (bm ybm (8) y =1 yb ybfo ) + (b yfo bf ybf ) (9) The impact of the estimation error becomes more clear if, instead of adding and subtracting ybfo , we instead add and subtract ym y f = (bm ybm = (bm ybm = (y m bfo my bm yb0 ) + (bm yb0 f f bm yb0 ) + bm f bm yb0 ) + (bm yb0 f f bf ybf ) bf yb0 + bf yb0 f f yf ) ybf (10) (11) Thus, the b terms contribute to both the explained and unexplained portions of the mean decomposition. In principle it is possible to separate out the e¤ect of gender di¤erences in the b parameter from the e¤ect of di¤erences in other parameters eq (10). However, this is 37 only feasible if the econometrician estimates both the bm and bf terms. In our case, we lack su¢ cient data to identify the weights in the model for females. Consequently, we only are able to decompose the di¤erence in mean outcomes into the portion caused by di¤erences in weights and di¤erences in characteristics according to eq (11). 38 REFERENCES Anderson, James M., Je¤rey R. Kling, and Kate Stith, “Measuring Interjudge Sentencing Disparity: Before and after the Federal Sentencing Guidelines,”Journal of Law and Economics, 1999, 42, 271–298. Becker, Gary, “Crime and Punishment: an Economic Approach,” Journal of Political Economy, 1968, 76, 169–217. Block, Michael K. and Vernon E. Gerety, “Some Experimental Evidence on Di¤erences Between Student and Prisoner Reactions to Monetary Penalties and Risk,” Journal of Legal Studies, 1995, 24, 123–138. Chicago Daily Law Bulletin, Law Bulletin Publishing Company, 27 December 2005. Defendants Sentenced Under the Guidelines During Fiscal Year 2001: SC01OUT, Washington, D.C.: Bureau of Justice Statistics, Federal Justice Statistics Program, 2001. Ehrlich, I, “Participation in Illegitimate Activities: a Theoretical and Empirical Investigation,”Journal of Political Economy, 1973, 81, 521–565. , “Capital Punishment and Deterrence: Some Further Thoughts and Additional Evidence,”Journal of Political Economy, 1977, 85, 741–788. Fairly, Robert W., “An Extension of the Blinder-Oaxaca Decomposition Technique to Logit and Probit Models,”Journal of Economic and Social Measurement, 2005, 30, 305–316. Freeman, Richard B, “Why do so Many Young American Men Commit Crimes and What Might we do about it?,”Journal of Economic Perspectives, 1999, 10, 25– 42. 39 Gould, Eric D., Bruce A. Weinberg, and David B. Mustard, “Crime Rates and Local Labor Market Opportunities in the United States: 1979-1997,”Review of Economics and Statistics, 2002, 84, 45–61. Greene, William H., Econometric Analysis, 3rd edition, New Jersey: Prentice-Hall, 2003. Grogger, Je¤rey, “The E¤ect of Arrests on the Employment and Earnings of Young Men,”Quarterly Journal of Economics, 1995, 110, 51–71. , “Market Wages and Youth Crime,”Journal of Labor Economics, 1998, 16, 756–791. Imai, Susumu and Kala Krishna, “Employment, Deterrence and Crime in a Dynamic Model,”International Economic Review, 2004, 45, 845–872. Johnson, Norman L. and Samuel Kotz, Distributions in Statistics: Continuous Multivariate Distributions, New York: John Wiley and Sons, 1972. Johnson, Ryan S., Shawn Kantor, and Price V. Fishback, “Striking at the Roots of Crime: the Impact of Social Welfare Spending on Crime During the Great Depression,”NBER Working Paper, 2007, No.12825. Kempf-Leonard, Kimberly and Lisa L. Sample, “Have Federal Sentencing Guidelines Reduced Severity? An Examination of one Circuit,” Journal of Quantitative Criminology, 2001, 17, 111–144. Kling, Je¤rey, “Incarceration Length, Employment and Earnings,”The American Economic Review, 2006, 96, 863–876. Kuziemko, Illyana, “Does the Threat of the Death Penalty A¤ect Plea Bargaining in Murder Cases? Evidence from New York’s 1995 Reinstatement of Capital Punishment,”American Law and Economics Review, 2006, 8, 116–142. 40 Levitt, Steven D., “Using Electoral Cycles in Police Hiring to Estimate the E¤ect of Police on Crime,”American Economic Review, 1997, 87, 270–290. Lott, John R. Jr., “An Attempt at Measuring the Total Monetary Penalty from Drug Convictions: The Importance of an Individual’s Reputation,” The Journal of Legal Studies, jan 1992, 21 (1), 159–187. Mustard, D.B., “Racial, Ethnic, and Gender Disparities in Sentencing: Evidence from the U.S. Federal Courts,”Journal of Law and Economics, 2001, 44, 285 –314. Myers, Samuel L. Jr., “Estimating the Economic Model of Crime: Employment Versus Punishment E¤ects,” The Quarterly Journal of Economics, feb 1983, 98 (1), 157–166. Neuman, Shoshana and Ronald L. Oaxaca, “Wage Decompositions with SelectivityCorrected Wage Equations: a Methodological Note,” Journal of Economic Inequality, 2004, 2, 3–10. New York Times, Justices to Revisit Thorny Issue of Sentencing Guidelines in First Case After Recess, Feb 20, 2007, Section A, 15. Oaxaca, Ronald L., “Male-Female Wage Di¤erentials in Urban Labor Markets,”International Economic Review, 1973, 14, 693–709. and Michael R. Ransom, “On Discrimination and the Decomposition of Wage Di¤erentials,”Journal of Econometrics, 1994, 61, 5–21. and Supriya Sarnikar, “Do Females Receive Lenient Sentences Despite the Federal Sentencing Guidelines?,”Mimeo, 2005. Schanzenbach, M., “Racial and Sex Disparities in Prison Sentences: the E¤ect of District-Level Judicial Demographics,”Journal of Legal Studies, 2005, 34, 57–92. 41 Tonry, Michael, Sentencing Matters, Oxford: Oxford University, 1996. Verdier, Thierry and Yves Zenou, “Racial Beliefs, Location, and the Causes of Crime,”International Economic Review, 2004, 45, 731–760. Waldfogel, Joel, “The E¤ect of Criminal Conviction on Income and the Trust ’Reposed in the Workmen,”The Journal of Human Resources, 1994, 29, 62–81. Witte, Ann D. and Pamela A. Reid, “An Exploration of the Determinants of Labor Market Performance for Prison Releasees,” Journal of Urban Economics, 1980, 8, 313–329. Witte, Ann Dryden, “Estimating the Economic Model of Crime with Individual Data,” The Quarterly Journal of Economics, 1980, 94, 57–84. Yun, Myeong-Su, “Generalized Selection Bias and the Decomposition of Wage Di¤erentials,”IZA Discussion Paper, 1999, Number 69. 42 Table 1 Percentage of Sentences Involving No Prison Time Year 1996 1997 1998 1999 2000 2001 2002 Total (%) 25.26 25.25 21.63 21.98 23.21 21.67 22.42 Males Trial (%) 6.45 4.83 4.56 9.76 4.78 9.90 5.63 Plea (%) 27.00 26.99 22.94 22.74 24.13 22.10 22.86 Total (%) 44.41 41.85 37.67 39.97 38.03 35.84 42.57 Females Trial (%) 12.73 21.74 18.60 34.88 10.26 14.81 17.39 Plea (%) 46.34 42.80 38.42 40.15 39.00 36.32 43.04 Table 2 Variable Definitions and Summary Statistics Variable TOTALMONTHS REGIME FEMALE FINEWAIV HISCHOOL GED SOMECOLL COLLGRAD NUMDEPEN MARRD CITIZN DEFENSEP XCRHISSR CRIMHIS1 CRIMHIS2 CRIMHIS3 CRIMHIS4 CRIMHIS5 CRIMHIS6 XFOLSOR XFOLSOR2 XFOLSOR3 AGE AGE2 CIRC1 CIRC2 CIRC3 CIRC4 CIRC5 CIRC6 CIRC7 CIRC8 CIRC9 CIRC10 CIRC11 Description Length of prison sentence in months Indicator for trial regime Indicator for female Indicator of fine being waived Indicator for high school education Indicator for general equivalency diploma Indicator for some college attended Indicator for a college degree or higher Number of dependents Indicator for married or cohabiting Indicator for US citizen Indicator for private counsel Final criminal history category Final criminal history category =1 Final criminal history category =2 Final criminal history category=3 Final criminal history category =4 Final criminal history category =5 Final criminal history category =6 Final offense level Final offense level squared Final offense level cubed Age of defendant Age of defendant squared Circuit indicators Circuit indicators Circuit indicators Circuit indicators Circuit indicators Circuit indicators Circuit indicators Circuit indicators Circuit indicators Circuit indicators Circuit indicators Overall Mean Std. Dev 37.27 73.12 0.05 0.22 0.18 0.38 0.84 0.37 0.24 0.43 0.13 0.34 0.26 0.44 0.12 0.32 1.13 1.41 0.26 0.44 0.95 0.21 0.36 0.48 2.11 1.60 0.58 0.49 0.12 0.32 0.13 0.33 0.06 0.24 0.04 0.19 0.08 0.27 16.80 8.30 351.02 325.02 8507.77 11499.56 37.74 11.14 1548.66 888.58 0.03 0.16 0.11 0.31 0.04 0.21 0.05 0.21 0.10 0.30 0.08 0.28 0.04 0.20 0.08 0.27 0.27 0.44 0.05 0.23 0.14 0.35 Males Mean Std. Dev 41.67 78.03 0.06 0.23 0.00 0.00 0.83 0.38 0.23 0.42 0.14 0.34 0.25 0.43 0.13 0.33 1.13 1.44 0.26 0.44 0.95 0.22 0.37 0.48 2.23 1.66 0.54 0.50 0.12 0.33 0.13 0.34 0.07 0.25 0.04 0.20 0.09 0.29 17.37 8.35 371.49 332.24 9140.23 11899.77 38.08 11.22 1576.29 900.04 0.03 0.17 0.12 0.32 0.05 0.21 0.05 0.21 0.10 0.30 0.08 0.27 0.04 0.20 0.08 0.27 0.27 0.44 0.05 0.22 0.14 0.35 Females Mean Std. Dev 16.76 37.07 0.03 0.18 1.00 0.00 0.87 0.34 0.28 0.45 0.11 0.32 0.29 0.45 0.07 0.26 1.09 1.29 0.25 0.43 0.97 0.16 0.30 0.46 1.57 1.16 0.74 0.44 0.09 0.29 0.10 0.29 0.03 0.17 0.02 0.13 0.03 0.16 14.11 7.51 255.55 269.16 5558.17 8832.43 36.16 10.59 1419.79 820.88 0.02 0.14 0.08 0.27 0.04 0.19 0.05 0.21 0.12 0.32 0.09 0.29 0.04 0.20 0.09 0.29 0.28 0.45 0.06 0.24 0.13 0.34 1996 1997 1998 1999 2000 2001 2002 Year indicators Year indicators Year indicators Year indicators Year indicators Year indicators Year indicators 0.13 0.13 0.13 0.14 0.14 0.15 0.17 0.33 0.34 0.34 0.35 0.35 0.36 0.37 0.13 0.14 0.13 0.14 0.14 0.15 0.17 0.33 0.34 0.34 0.34 0.35 0.36 0.38 0.12 0.13 0.14 0.15 0.15 0.15 0.16 0.33 0.34 0.35 0.36 0.35 0.36 0.36 Table 3 Mean Sentences in Months Year 1996 1997 1998 1999 2000 2001 2002 Total 43.26 43.81 42.00 42.15 40.12 41.20 39.87 Males Trial 133.44 136.47 124.29 112.62 108.01 94.50 111.85 Plea 34.92 35.93 35.66 37.79 36.75 39.24 38.00 Total 15.00 19.27 16.07 15.08 15.93 18.27 17.58 Females Trial 44.37 94.50 36.17 30.66 44.85 59.19 55.26 Plea 13.21 15.73 15.28 14.51 14.92 17.34 16.88 Table 4 Censored Switching Regression with Endogenous Switching: Pooled Sample Variable Constant FEMALE FINEWAIV HISCHOOL GED SOMECOLL COLLGRAD CITIZN MARRD NUMDEPEN DEFENSEP CRIMHIS2 CRIMHIS3 CRIMHIS4 CRIMHIS5 CRIMHIS6 XFOLSOR XFOLSOR2 XFOLSOR3 AGE AGE2x10-2 CIRC2 CIRC3 CIRC4 CIRC5 CIRC6 CIRC7 CIRC8 CIRC9 CIRC10 CIRC11 1996 1997 1998 1999 2000 2001 Sigma 0 Rho 0u Sigma 1 Rho 1u N Log-Likelihood Regime Selection Parameter Asmp Z -2.685 -17.17 -0.198 -5.90 0.001 0.020 0.017 0.186 -0.043 0.053 -0.008 -0.039 0.038 0.030 0.019 0.107 0.077 0.03 0.57 0.55 4.88 -1.65 1.67 -0.97 -1.72 1.12 0.87 0.39 1.93 2.19 0.035 -0.024 -0.385 -0.252 -0.160 -0.266 -0.039 -0.023 -0.217 -0.242 -0.184 0.003 0.535 0.506 0.464 0.348 0.258 0.128 4.91 -2.79 -5.92 -3.37 -2.14 -3.98 -0.59 -0.31 -3.30 -4.04 -2.58 0.05 12.04 11.45 10.28 8.05 5.80 2.82 47.109 -0.663 215.842 0.994 45060 -189907.6 958.51 -67.95 102.38 1692.69 Trial Regime Parameter Asmp Z -768.983 -17.66 -48.700 -5.67 3.944 0.80 -3.030 -0.41 4.163 0.49 0.882 0.12 30.596 3.31 10.319 -2.081 -9.220 22.950 29.251 39.424 76.201 74.597 13.632 -0.480 0.009 8.396 -6.123 -98.366 -50.159 -30.082 -60.598 -3.004 6.603 -36.302 -53.839 -34.254 7.136 123.182 115.919 104.472 71.825 56.937 25.239 2333 1.38 -1.12 -1.66 2.82 3.50 3.44 5.84 9.53 5.16 -4.73 7.43 4.93 -2.99 -6.30 -2.75 -1.70 -3.79 -0.19 0.36 -2.29 -3.68 -2.02 0.49 11.53 11.12 9.68 7.04 5.38 2.29 Plea Regime Parameter Asmp Z -128.508 -30.91 -9.223 -10.29 4.695 5.73 -1.105 -1.50 1.192 1.53 -1.519 -2.20 -1.836 -1.73 -2.715 -0.873 -5.525 8.531 18.795 30.676 38.444 53.103 15.799 -0.723 0.014 0.249 -0.430 -6.391 1.783 12.412 10.880 8.319 17.144 -1.485 2.105 3.417 10.045 -0.603 -0.030 0.529 1.511 1.800 1.759 42727 -4.02 -4.55 -9.48 9.04 24.54 29.92 27.52 69.13 51.80 -53.66 79.57 1.45 -2.01 -3.24 0.79 5.70 5.49 4.00 7.76 -0.76 1.13 1.57 5.30 -0.56 -0.03 0.47 1.49 1.78 1.80 Table 5 Censored Switching Regression with Endogenous Switching: Males Variable Constant FINEWAIV HISCHOOL GED SOMECOLL COLLGRAD CITIZN MARRD NUMDEPEN DEFENSEP CRIMHIS2 CRIMHIS3 CRIMHIS4 CRIMHIS5 CRIMHIS6 XFOLSOR XFOLSOR2 XFOLSOR3 AGE AGE2x10-2 CIRC2 CIRC3 CIRC4 CIRC5 CIRC6 CIRC7 CIRC8 CIRC9 CIRC10 CIRC11 1996 1997 1998 1999 2000 2001 Sigma 0 Rho 0u Sigma 1 Rho 1u N Log-Likelihood Regime Selection Parameter Asmp Z -2.638 -15.64 0.017 0.036 0.025 0.171 -0.042 0.055 -0.006 -0.049 0.033 0.041 0.035 0.113 0.077 0.51 0.94 0.77 4.18 -1.52 1.60 -0.70 -1.96 0.89 1.13 0.71 1.94 2.11 0.034 -0.024 -0.397 -0.258 -0.166 -0.278 -0.074 -0.034 -0.246 -0.259 -0.176 -0.013 0.538 0.514 0.481 0.357 0.257 0.128 4.46 -2.64 -5.80 -3.26 -2.10 -3.89 -1.06 -0.43 -3.52 -4.08 -2.33 -0.21 11.14 10.70 9.72 7.65 5.34 2.62 49.543 -0.692 224.546 0.994 37104 -163499.9 858.88 -72.20 93.54 1535.52 Trial Regime Parameter Asmp Z -767.458 -15.67 3.340 0.60 -1.423 -0.17 7.070 0.75 1.778 0.21 26.557 2.58 11.390 -1.706 -11.229 22.167 32.000 43.492 78.039 76.024 11.693 -0.404 0.008 8.507 -6.336 -103.768 -52.720 -33.349 -61.521 -9.518 4.737 -42.872 -57.704 -30.395 4.682 126.383 120.490 111.016 75.419 55.756 24.521 2057 1.35 -0.83 -1.80 2.42 3.49 3.50 5.39 9.01 3.94 -3.54 5.97 4.43 -2.76 -6.08 -2.63 -1.71 -3.49 -0.53 0.24 -2.44 -3.59 -1.63 0.29 10.40 10.17 8.99 6.58 4.69 1.99 Plea Regime Parameter Asmp Z -128.041 -26.57 5.149 5.52 -1.611 -1.88 1.172 1.32 -1.885 -2.37 -1.521 -1.28 -3.354 -0.948 -6.532 8.394 19.239 31.494 38.609 53.490 15.581 -0.699 0.014 0.185 -0.343 -6.478 1.005 12.327 9.425 7.088 15.882 -1.560 1.188 2.539 9.672 -1.064 -0.825 -0.272 1.409 1.362 1.539 35047 -4.30 -4.33 -9.74 7.75 21.88 27.17 24.59 61.44 43.19 -44.14 65.83 0.94 -1.40 -2.89 0.39 4.94 4.14 2.98 6.31 -0.69 0.56 1.01 4.48 -0.85 -0.68 -0.21 1.19 1.16 1.36 Table 6 Mean Sentences and Conviction-by-Trial Probabilities Variable ȳ ȳ T ȳ P p̄ T ̂ T ̂ P ̂ s ̂ Males 41.673 120.845 37.027 0.055 0.852 0.992 0.894 0.962 Females 16.757 51.736 15.500 0.035 Difference 24.916 69.109 21.526 0.021 Male Fitted 43.320 141.770 37.320 0.062 Females Fitted (Male Weights) 25.737 89.281 22.776 0.051 Table 7 Decomposition by Part Variable ȳ Tm − ȳ Tf ȳ Pm − ȳ Pf p̄ Tm − p̄ Tf Explained Unexplained Total Gap 31.564 37.545 69.109 14.251 7.275 21.527 0.004 0.016 0.021 Table 8 Contribution to Total Explained E T p̄ Tm E P 1 − p̄ Tm E s ȳ Tf − ȳ Pf E Unexplained 1.750 13.461 0.159 15.370 U T p̄ Tm U P 1 − p̄ Tm U s ȳ Tf − ȳ Pf U Total Gap 2.081 6.872 0.593 9.546 E T p̄ Tm U T p̄ Tm E P 1 − p̄ Tm U P 1 − p̄ Tm E s ȳ Tf − ȳ Pf U s ȳ Tf − ȳ Pf EU 3.831 20.333 0.752 24.916